Lost in Translation: The Challenge of Teaching AI Human Languages
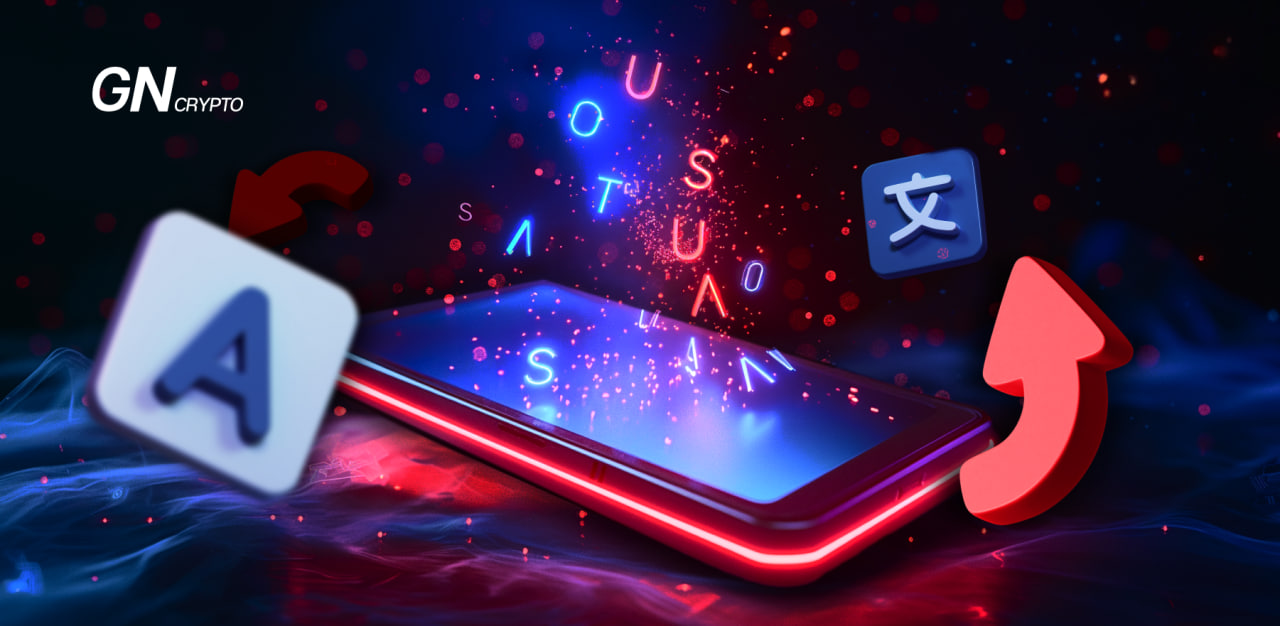
There are around 4,000 languages written in the world according to Ethnologue. The maximum number of languages that AI-powered apps like Google Translate and ChatGPT support is below 150. These AI tools are not equally fluent in all languages. Since they are trained on vast amounts of data in English, they understand this language the best.
English is like the native language of AI. Yet, the language AI understands and communicates lacks emotional colors and a distinctive tone of voice. Although AI systems are learning to recognize human emotions, there are many nuances they are still far from detecting even in English.
For example, you can ask ChatGPT to write something sarcastic, and it may come up with some witty answer, but it won't understand your jokes as a human might. In the multicultural dimension, the limitations become more noticeable. To make sense of this, below is the breakdown of how AI understands human languages and what challenges it faces.
Communication Between Computers and Human Languages
The technology that empowers computers to interact with human languages is called Natural Language Processing (NLP). It has been developed as a result of the collaboration between computer science and linguistics. NLP is focused on building computational models that can understand, analyze, and generate responses to human language.
Companies in the technology field use NLP to train their AI applications. When you see an AI language learning chatbot, speech-to-text converter, voice recognition, and other apps related to speech and languages, NLP is a part of it. The technology is foundational to the functionality of Google Translate, Apple’s Siri, Facebook's personalized recommendations, OpenAI’s GPT language model, etc.
NLP has been a research field in AI for decades. With the advent of Machine Learning, it empowers AI systems to learn from datasets that include large amounts of words and translations. Due to constant training and improvement, AI language models are getting better and better. Google Translator is a good example. The app now better understands context and translates more accurately than years ago. This is said both by user reviews and company updates.
Despite the progress made, AI systems still face the challenge of accurately translating words. Apps can go wrong, especially when dealing with words that express cultural components or have several meanings. A common blunder among AI apps is translating names of places or traditions that don't require translation. Sometimes, translations just don’t have any point. They can look like a group of words randomly put together.
To solve the gap, tech companies have been working on multilingual language models. The concept of this technology is to train on data not only in one language but to use text from multiple languages at the same time. Doing so helps machines spot connections and patterns between languages, to achieve better results.
Low-Quality Translations Flooding the Web
As we mentioned computers' linguistic abilities are limited, but it’s the human factor after all that decides what to do with limitations and how to use the technologies. It’s up to people whether to improve translations and provide quality content to the audience or to take AI output and use it without checking. According to recent research by the University of California and Amazon Web Services AI Lab, a shocking amount of the web is machine-translated. The paper mentions:
Content on the web is often translated into many languages, and the low quality of these multi-way translations indicates they were likely created using Machine Translation (MT).
The picture is particularly disappointing for low-resource languages which are languages with little amount of content available on the internet. It was found by the same research that machine-generated translations in low-resource languages make up a large fraction of total web content in those languages. The goal for these translations is supposed to be for profit. Based on investigation, first, poor-quality content is generated in English likely to generate ad revenue, and then translated en masse into many lower-resource languages through Machine Translation.
The low-quality translations make it more difficult for AI to learn languages. Because large language model training includes web-scraped data, poor-quality content can in turn result in incorrect data training for the systems.
Can AI Get Better in Human Languages?
AI systems today memorize millions of words. They are good enough to help people communicate using different languages, a capability appreciated by travelers, for example. Language abilities of AI are improving along with technological progress, like the improvement of multilingual language models. But, at the same time, there are new challenges, including faulty and low-quality content on the web. That being said, AI’s path to mastering human languages is quite complex. What will remain lost in translation and what challenges the technology will be able to deal with, is yet to be seen.