What is an AI Hallucination? Exploring the Phenomenon
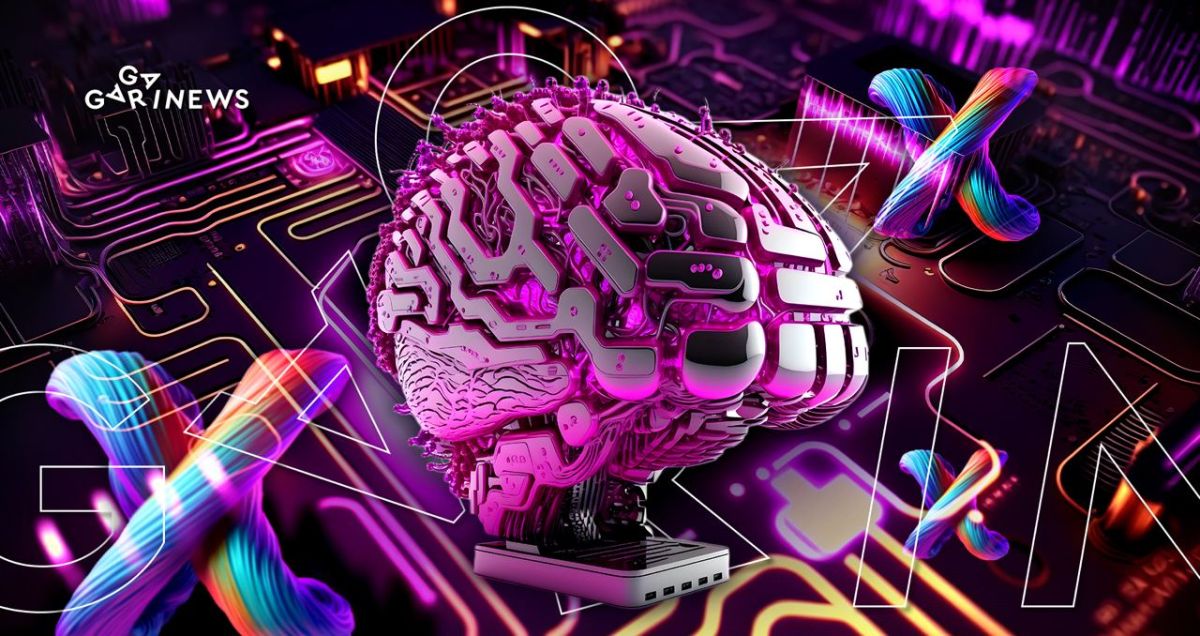
AI hallucinations represent the instances where a deep learning model's internal structure generates erroneous results that cannot be backed up by the input data. These could take the form of an image portraying a non-existent entity, a simulated musical piece, or a text that appears realistically structured but is in fact nonsensical.
Even with the widespread success and adoption of artificial intelligence across diverse fields such as technology, medicine, and the arts, these logical blunders remain a major hurdle for developers. They can lead to unforeseeable outcomes and incite skepticism among users regarding the dependability of emerging technologies.
What Triggers AI Hallucinations?
These aberrations primarily stem from the complex operations within multi-layered neural network structures. These structures consist of artificial neurons that mimic the function of real cells, but in the form of a non-linear function. Throughout the training phase, algorithms sift through the input data, attempting to identify patterns and assign a specific significance to each neuron. Consequently, information filters through numerous networks, becoming more abstract and enabling the creation of fresh content based on the learning materials. However, occasional errors emerge that negatively alter the outcome. Researchers have yet to pinpoint the precise causes of this phenomenon, but they have proposed several plausible explanations:
1. Overfitting: In instances of specialized training or excessive adaptation to the source data, models may generate hallucinations when faced with unfamiliar or ambiguous requests.
2. Bias: If the initial data set predominantly leans in a particular direction, the artificial intelligence may churn out incorrect responses due to a considerable shift in the data balance.
3. Insufficient training: To thoroughly understand complex patterns and deliver accurate results, a comprehensive database is necessary. A lack thereof could trigger the model to induce hallucinations.
4. Excessive training: An information overload could instigate distortions, as the increased noise levels reduce the importance of rare neurons, causing discrepancies.
5. Model architecture: The existence of numerous parameters can influence the likelihood of hallucinations, and hyperparameters (such as noise regulation methods, diversity level of responses) can enhance their occurrence.
6. Lack of context comprehension: Often, training models fail to grasp the context and incorrectly interpret the relationships between objects within the input data, leading to information distortion.
7. Technical validation of results: Artificial intelligence invariably relies on software analysis to verify its functioning, an approach that may not always yield the desired response for a human user.
Certainly, this is merely a tentative list of theories. Once the final output has been generated, it's practically impossible to pinpoint the internal processes that guided the model to its conclusion. For instance, ChatGPT formulates its text based on user input and previously formed phrases. If it generates a hallucination once, the chatbot may subsequently increase the amount of incorrect data in a proportional manner.
There are still many cases where you ask ChatGPT a question and it'll give you a very impressive-sounding answer that's just dead wrong. And, of course, that's a problem if you don't carefully verify or corroborate its facts,- © Oren Etzioni, the CEO and founder of the Allen Institute for AI.
With this in mind, OpenAI is taking steps to tackle the problem and is warning users about the possibility of the system generating incorrect answers. Other AI development companies are also fully aware of these so-called hallucinations. They are calling on researchers worldwide to help reduce these distortions, as no concrete solution has been found so far. A thorough examination of this problem could yield essential insights into AI operations, aiding future advancements.
What is an AI hallucination and how does it impact the accuracy of generated content?
An AI hallucination is a phenomenon where an artificial intelligence system generates content that appears logical and coherent but is factually incorrect or entirely fabricated. This occurs because the AI often relies on patterns from its training data, which can sometimes lead to the production of false information. AI hallucinations impact the accuracy of generated content by potentially spreading misinformation, leading users to make decisions based on inaccurate data. This is particularly concerning in critical applications such as medical diagnosis, legal advice, or news reporting, where factual accuracy is paramount.